Bayesian Theory and the Theory of Life
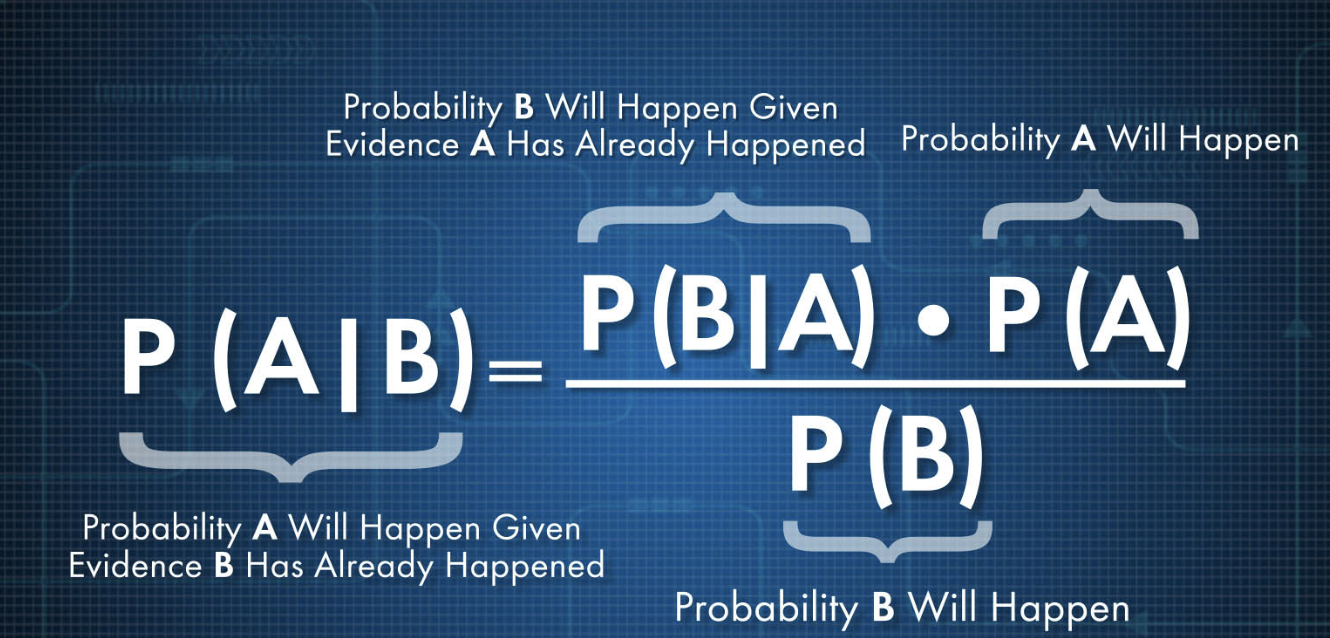
One of the reasons I love working in Manhattan is all the cool opportunities to learn and the networks of interesting people. Every Monday I receive an email from tech investor Charlie O’Donnell with classes a list of classes and meetups for entrepreneurs going on around New York City.
Last week I found out and signed up for a high level class on Bayesian Theory and probability (called Bayesian Theory and the Theory of Life) that met during lunch time a few blocks from my office. The class offered through Skillshare (interesting but seemingly immature startup) and the instructor was Albert Wegner, a partner with venture capital firm Union Square Ventures.
I am someone who generally questions a lot of accepted hypotheses, baselines, and beliefs. So I was interested to see this presentation how we could use Bayes Theory to test whether a prior belief is correct in relation to what we measure.
His agenda was written on the white board as follows:
- Why does uncertainty exist?
- Why do we suck at dealing with #1?
- How do we think correctly about uncertainty?
- What does it mean for life?
Some Background Exercises and Concepts
So Wegner started the class having us answer some multiple choice and open ended questions, and they helped to illustrate some excellent points:
- The source of uncertainty is limited knowledge of the world. Can we ever really be certain about anything?
o Maybe we can have certainty for really small systems. Even then it is very likely there is some level of the unknown that could affect our certainty of an outcome.
o We also need to consider how the cost of observations affects our certainty. Are we sacrificing something important to make those observations?
o We also need to consider how the speed at which we can make observations affects our certainty. Can we make and process observations quickly enough for them to be relevant?
o Quantum uncertainty – if we observer something too closely we may change what you are trying to measure.
- One of the scenarios described an introverted shy, nerdy individual and then asked which was a more likely career path – librarian or truck driver.
The correct answer was truck driver, because there are 1.5 million of those positions available vs. 150,000 librarians. Even if there is a possible social predisposition, it would be difficult to assume that it would weigh greater than the 10x multiplier difference of the population dynamics.
This concept is referred to as the Base Rate Fallacy.
- He gave an example that the Bill Gates Foundation did a study that the best schools in the US were small schools, the foundation and government gave small schools lots of money. He then commented that small schools also accounted for the worst schools, while larger schools seemed to have a more middle of the road position.
He noted that the law of small numbers could be in play here, as analyzing small populations can lead to misleading extremes. It is very popular a small school could have a majority of either good or bad students. The larger populations will have both good and bad averaged together, accounting for a blended score.
- My favorite question was “Intelligent women tend to marry less intelligent men. Why?” The asked this to half the class, and the other have had intelligent men with less intelligent women.
He drew the following diagram on the board for us:
In a fairly common linear regression scenario, we can draw a line that that shows the trend for all the individual data points. He then drew an oval around the data points. He explained that as the smartness of woman got to the higher end, the number of men under the line in the oval increased proportionally. The inverse would be true as the smartness of men increased.
He mentioned that smartness of men and woman are imperfectly correlated – meaning that they do not go up together and one may go down as the other goes up.
- We don’t observe probability distributions, only outcomes. So we try to tie our expectations about uncertainty to outcomes.
- 2 types of modes our brain works in:
o Type 1 – assumptions and thought processes we just make automatically
o Type 2 – we knowingly realize we need deeper analysis, and take a step back to do the analysis
His explanation Bayesian Theory
Bayes Theory is about testing hypothesis against the observed outcomes.
He gives an example about home HIV tests. Here are the numbers we’ll dive into:
- The US population had 309 million people and 1.2 million had HIV (0.3%).
- The home test is 99.8% accurate in measuring people who don’t have HIV.
- The home test is 92% accurate in measuring people who do have HIV.
1 million people
|
|
------------------------------------------------------------
3,000 | | 997,000
| |
HIV NO HIV
| |
| |
----------------- -----------------
92% | | 8% 0.2% | | 99.8%
| | | |
2,760 240 1,994 995,006
+ - + -
- The 995,006 have correctly tested negative
- The 1,994 have tested false positive
- The 240 have tested false negative
- The 2,760 have correctly tested positive
E=Evidence, H=Hypothesis, P=Probability
|
E |
!E |
H |
P(H&E) |
P(H&!E) |
!H |
P(!H%E) |
P(!H&!E) |
P(H) = 0.3%
(the hypothesis or prior belief)
P(H|E) = 92%
(probability of hypothesis given evidence observed)
P(E) = P(H&E) + P(!H%E) = 2760+1994 = 4754
(total of expected positive with false positive)
P(H&E) / P(E) = 2760/(2760+1994) = 58%
(divide expected positive by total of expected positive with false positive)
Our goal is to calculate the probability that the hypothesis is accurate, given the fact that the evidence observed. Using the formula for Bayes' theorem, we have:
Bayes Theorum:
P(E|H) / P(E) * P(H) = .92 / .04754 * .0003 = .0058
(divide the probability of hypothesis by expected positive the divide by total of expected positive with false positive and then multiply by the hypothesis)
Any time P(E|H) is greater than P(E), you likely have a good test that will provide lift.
In this case the 0.58% of total people attributed to having HIV is increased compared to the hypothesis of 0.3%.
Strong prior belief leads to lesser updating. People tend to hold beliefs and signals (tests) will be less impactful.
My take on the class
I very much enjoyed the course content and my refresher on probability (it has been 15+ years since college since I had classes covering probability). The instructor did a good job with the background exercises, as they did a good job illustrating the concepts.
I would have liked him to spend a little more time explaining the value of Bayes Theory before jumping into the scenario. It became a little confusing (even for the instructor) whether the HIV test example represents a good (reliable) test based on the statistics.
I came out of the class thinking it was about validating a hypothesis, but I see it now being more about understanding the relationship between correlated probabilities.
In the last 2 minutes of the class he was trying to tie in the discussion of the class to how we can deal with life's uncertainties. I thought it was a bit of a stretch, but it was entertaining.
Applying it to one of my projects:
After the class, I read up on Bayes Theorum a little bit online and found some descriptions a little easier for me to understand.
The Problem
I am interested in how visitation relates to conversion rates between free membership and paid subscriptions for SportsCollectors.Net.
Using Bayes to figure it out
- Out of 33,000 registered users, 6,842 have subscribed (20.7%)
- Members that visit the site 50 or more times become subscribers 74% of the time.
- Members that the site less than 50 times become subscribers 8% of the time.
P(S) = Probability of member subscription = .207
P(A) = Probability member has 50+ visits = .23
P(S|A) = Probability subscriber is a member with 50+ visits = .74
P(B) = Probability member has under 50 visits = .77
P(S|B) = Probability subscriber is member with under 50 visits = .08
P(A|S) = P(A)P(S|A) .23 * .74 .1702
---------------------------------- = ---------------------------- = ------------ = .7343
P(A)P(S|A) + P(B)P(S|B) .23 * .74 + .77 * .08 .2318
The probability of a person visiting the site 50+ times is going to buy a subscription is at 73%.
P(B|S) = P(B)P(S|B) .77 * .08 .01816
---------------------------------- = ---------------------------- = ------------ = .2657
P(B)P(S|B) + P(S)P(S|A) .77 * .08 + .23 * .74 .2318
The probability of a person visiting the site less than 50 times is going to buy a subscription is under 27%.
Analysis:
From these numbers, I’m going to consider increasing my attention to getting non premium members to visit the site more regularly (with freemium features) if a goal is to convert them to paying subscribers.
I am not sure it is realistic to expect a new user to visit the site 50 times. I will re-run the procedure with thresholds of 10, 20 ,and 25, 35 visits to see how the relationship of visitation on subscription rates changes.
Pseudo SQL Code that could be used to get this info:
1
2
3
4
5
6
7
8
9
10
11
12
13
14
15
16
17
18
19
20
21
22
23
24
25
26
27
28
29
30
31
32
33
34
35
36
37
38
39
40
41
42
43
44
45
46
47
48
49
50
51
52
53
54
DECLARE @threshold int
DECLARE @TotalUsers int
DECLARE @TotalSubcribers int
DECLARE @SubcriptionProbability decimal(5,2)
DECLARE @TotalUsersAboveThreshold int
DECLARE @TotalSubcribersAboveThreshold int
DECLARE @SubcriptionProbabilityAboveThreshold decimal(5,2)
DECLARE @TotalUsersBelowThreshold int
DECLARE @TotalSubcribersBelowThreshold int
DECLARE @PercentUsersAboveThreshold decimal(5,2)
DECLARE @PercentUsersBelowThreshold decimal(5,2)
DECLARE @SubcriptionProbabilityBelowThreshold decimal(5,2)
DECLARE @ProbablityAboveThresholdWillSubscribe decimal(5,2)
DECLARE @ProbablityBelowThresholdWillSubscribe decimal(5,2)
SET @threshold=50
SET @TotalUsers=(SELECT COUNT(USERID) From UserInfo)
SET @TotalSubcribers=(SELECT COUNT(USERID) From UserInfo WHERE IsPremium = true)
SET @SubcriptionProbability=CAST(@TotalSubcribers AS decimal)/CAST(@TotalUsers ASdecimal)
SET @TotalUsersAboveThreshold=(SELECT COUNT(USERID) From UserInfo WHERE VisitCount >=@Threshold)
SET @PercentUsersAboveThreshold=CAST(@TotalUsersAboveThreshold ASdecimal)/CAST(@TotalUsers AS decimal)
SET @TotalSubcribersAboveThreshold=(SELECT COUNT(USERID)From UserInfo WHERE IsPremium = true AND VisitCount >= @Threshold)
SET @SubcriptionProbabilityAboveThreshold=CAST(@TotalSubcribersAboveThreshold ASdecimal)/CAST(@TotalUsersAboveThreshold AS decimal)
SET @TotalUsersBelowThreshold=(SELECT COUNT(USERID) From UserInfo WHERE VisitCount <@Threshold)
SET @PercentUsersBelowThreshold=CAST(@TotalUsersBelowThreshold ASdecimal)/CAST(@TotalUsers AS decimal)
SET @TotalSubcribersBelowThreshold=(SELECT COUNT(USERID)From UserInfo WHERE IsPremium = true AND VisitCount < @Threshold)
SET @SubcriptionProbabilityBelowThreshold=CAST(@TotalSubcribersBelowThreshold ASdecimal)/CAST(@TotalUsersBelowThreshold AS decimal)
SET @ProbablityAboveThresholdWillSubscribe=(@PercentUsersAboveThreshold*@SubcriptionProbabilityAboveThreshold)/((@PercentUsersAboveThreshold*@SubcriptionProbabilityAboveThreshold)+((1.00-@PercentUsersAboveThreshold)*@SubcriptionProbabilityBelowThreshold))
SET @ProbablityBelowThresholdWillSubscribe=(@PercentUsersBelowThreshold*@SubcriptionProbabilityBelowThreshold)/((@PercentUsersBelowThreshold*@SubcriptionProbabilityBelowThreshold)+((1.00-@PercentUsersBelowThreshold)*@SubcriptionProbabilityAboveThreshold))
PRINT 'Threshold: ' + CAST(@Threshold AS varchar(255))
PRINT 'TotalUsers: ' + CAST(@TotalUsers AS varchar(255))
PRINT 'TotalSubcribers: ' + CAST(@TotalSubcribers AS varchar(255))
PRINT 'SubcriptionProbability: ' + CAST(@SubcriptionProbability AS varchar(255))
PRINT ' '
PRINT 'TotalUsersAboveThreshold: ' + CAST(@TotalUsersAboveThreshold AS varchar(255))
PRINT 'PercentUsersAboveThreshold: ' + CAST(@PercentUsersAboveThreshold ASvarchar(255))
PRINT 'TotalSubcribersAboveThreshold: ' + CAST(@TotalSubcribersAboveThreshold ASvarchar(255))
PRINT 'SubcriptionProbabilityAboveThreshold: ' +CAST(@SubcriptionProbabilityAboveThreshold AS varchar(255))
PRINT ' '
PRINT 'TotalUsersBelowThreshold: ' + CAST(@TotalUsersBelowThreshold AS varchar(255))
PRINT 'PercentUsersBelowThreshold: ' + CAST(@PercentUsersBelowThreshold ASvarchar(255))
PRINT 'TotalSubcribersBelowThreshold: ' + CAST(@TotalSubcribersBelowThreshold ASvarchar(255))
PRINT 'SubcriptionProbabilityBelowThreshold: ' +CAST(@SubcriptionProbabilityBelowThreshold AS varchar(255))
PRINT ' '
PRINT '@ProbablityAboveThresholdWillSubscribe=(@PercentUsersAboveThreshold*@SubcriptionProbabilityAboveThreshold)/((@PercentUsersAboveThreshold*@SubcriptionProbabilityAboveThreshold)+((1.00-@PercentUsersAboveThreshold)*@SubcriptionProbabilityBelowThreshold))'
PRINT CAST(@ProbablityAboveThresholdWillSubscribe AS varchar(255)) + '=('+CAST(@PercentUsersAboveThreshold AS varchar(255)) + '*' +CAST(@SubcriptionProbabilityAboveThreshold AS varchar(255)) + ')/((' +CAST(@PercentUsersAboveThreshold AS varchar(255)) +'*'+CAST(@SubcriptionProbabilityAboveThreshold AS varchar(255)) + ')+((1.00-' +CAST(@PercentUsersAboveThreshold AS varchar(255)) + ')*' +CAST(@SubcriptionProbabilityBelowThreshold AS varchar(255)) + '))'
PRINT 'ProbablityAboveThresholdWillSubscribe: ' +CAST(@ProbablityAboveThresholdWillSubscribe AS varchar(255))
PRINT 'ProbablityBelowThresholdWillSubscribe: ' +CAST(@ProbablityBelowThresholdWillSubscribe AS varchar(255))